Learn about the 10 most discussed Sub-Topics in Artificial Intelligence đ§Ÿ
- Boris Thienert
- 1. Apr.
- 16 Min. Lesezeit
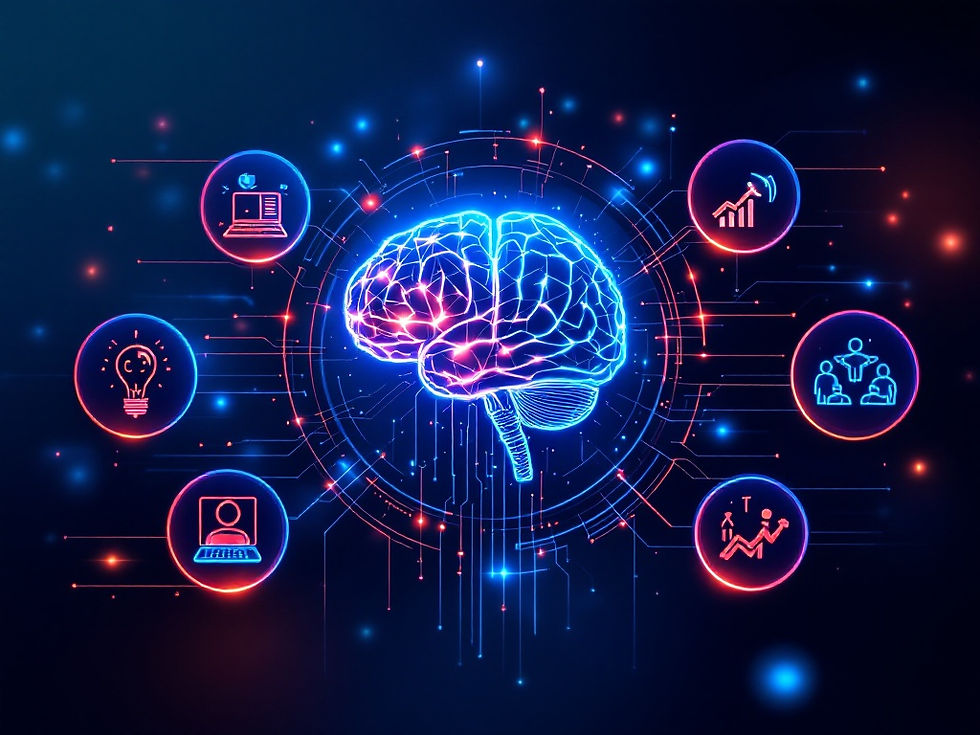
Introduction
Artificial Intelligence (AI) encompasses a broad range of technologies designed to
simulate human intelligence, enabling machines to learn, reason, and perform tasks
autonomously. Among its most discussed sub-topics in artificial intelligence such as
machine learning, natural language processing, computer vision, robotics,
neural networks, deep learning, AI ethics, autonomous systems, AI in healthcare and AI in finance.
Each of these areas is notable for its transformative impact across various industries,
reshaping how we interact with technology and manage complex data-driven challenges.
Machine learning, as a subset of AI, has gained significant traction due to its ability
to extract insights from vast datasets, making it a crucial skill for data science professionals.
Natural language processing allows for meaningful interactions between
computers and human language, enhancing applications in customer service and
healthcare. Similarly, computer vision plays a vital role in enabling machines to
interpret visual information, influencing sectors from security to autonomous vehicles.
Robotics merges AI with engineering to create systems capable of performing tasks
in diverse environments, further pushing the boundaries of automation.
Despite the advancements, each sub-topic faces its own set of challenges and
controversies. Machine learning and AI ethics grapple with issues of bias and transparency,
while autonomous systems raise critical questions about decision-making in
high-stakes scenarios. The integration of AI in healthcare and finance presents both
opportunities for enhanced efficiency and risks associated with privacy and ethical
considerations. As these fields evolve, ongoing discourse surrounding their implications
is essential to ensure responsible development and application in society.
Overall, the discourse around these ten prominent sub-topics underscores the importance
of AI in driving innovation while highlighting the need for ethical frameworks
and safeguards to navigate the complexities inherent in this rapidly advancing field.
10 very strong and important sub-topics in artificial intelligence are:
Machine Learning
Machine learning (ML) is a prominent subfield of artificial intelligence (AI) that
enables computers to learn from data without being explicitly programmed to perform
specific tasks.[1][2] As one of the most valuable skills for data science professionals in
2024, machine learning is becoming increasingly essential as its applications expand
across various industries.[3]
Evolution of Machine Learning
According to a report from Gartner, the landscape of machine learning is evolving
from simple predictive algorithms to a more data-centric discipline. This evolution signifies
a shift towards utilizing extensive datasets to drive insights and decision-making
processes, enhancing the overall effectiveness of machine learning applications.[4]
Practical Applications and Case Studies
Machine learning is often illustrated through case studies that analyze real-world
business challenges, demonstrating how ML techniques can be applied to solve
problems and provide valuable insights. Engaging with these case studies is crucial
for aspiring data scientists, as it showcases their practical expertise and bolsters their
resumes by highlighting hands-on experience with machine learning applications.[4]
Key Areas and Techniques
The field of machine learning encompasses several specialized areas, including
large-scale machine learning, deep learning, reinforcement learning, robotics, computer
vision, natural language processing, and recommender systems. Each of these
domains employs various algorithms and techniques tailored to specific types of data
and tasks, further broadening the impact of machine learning in different sectors.[4]
Importance in Data Science
As organizations increasingly prioritize data-driven decision-making, machine learning
is becoming integral to the skill sets required in the data science field. Employers
frequently seek candidates who possess not only technical capabilities but also
business acumen and data-handling skills. Therefore, working on real-world machine
learning projects can significantly enhance a professional's employability and marketability
in an ever-evolving job landscape.[4]
Natural Language Processing
Natural Language Processing (NLP) is a crucial subfield of artificial intelligence that
focuses on the interaction between computers and human language. It enables machines
to interpret, understand, and generate human language in a meaningful way.
NLP has seen significant advancements and applications across various domains.
Key Technologies
NLP employs several core technologies to process and analyze human language
effectively:
Tokenization: The process of breaking down text into individual words or phrases,
which serves as a foundational step for further analysis[5].
Part-of-Speech Tagging: This technique assigns grammatical categories to words,
such as nouns, verbs, or adjectives, facilitating better comprehension of sentence
structure[5].
Named Entity Recognition: This involves identifying and classifying key entities within
text, such as names of people, organizations, or locations[5].
Machine Translation: The automatic translation of text from one language to another,
which has improved significantly with the advent of deep learning techniques[5].
Applications
The applications of NLP are vast and impactful, particularly in sectors like healthcare
and customer service:
Healthcare: NLP enhances diagnostic accuracy by extracting pertinent information
from medical records and aiding clinicians in managing complex data efficiently. It
also identifies relevant treatments and predicts potential health risks, transforming
patient care and medical processes[6].
Customer Support: NLP powers chatbots and question-answering systems that provide
customers with accurate and coherent responses, streamlining communication
and improving user experience[7][8].
Challenges and Research Directions
While NLP has made remarkable strides, it still faces several challenges:
Understanding Nuance: Developing systems that can grasp the subtleties and context
of natural language remains a primary research focus, as nuanced understanding
is crucial for generating accurate responses[7][9].
Multimodal Integration: Bridging the gap between visual and textual data is an
emerging research area, particularly in generating descriptive captions for images,
which can enhance accessibility for visually impaired individuals and improve multimedia
indexing[7][9].
NLP continues to evolve, driven by ongoing research and advancements in AI technologies,
leading to improved capabilities in understanding and generating human
language.
Computer Vision
Computer Vision is a pivotal area within the field of Artificial Intelligence (AI) that
focuses on enabling machines to interpret and make decisions based on visual data.
This discipline encompasses various applications, techniques, and challenges that
drive research and development.
Definition and Technologies
Computer Vision involves the automatic extraction, analysis, and understanding of
useful information from a single image or a sequence of images. Key technologies
utilized in this domain include image processing, object detection, and pattern
recognition[10][11]. Notably, advancements in algorithms and computational power
have significantly propelled the capabilities of computer vision systems.
Key Applications
Computer Vision finds applications across a wide range of industries, including:
Facial Recognition: Employed in security systems and user authentication, facial
recognition technology analyzes facial features to identify individuals[10][12].
Autonomous Vehicles: Vehicles equipped with computer vision systems can interpret
their surroundings, enabling safe navigation and obstacle avoidance[11][13].
Medical Image Analysis: In healthcare, computer vision assists in diagnosing diseases
through the analysis of medical images such as X-rays and MRIs[11][14].
Surveillance: Enhancing security measures, computer vision systems can monitor
and analyze live video feeds to detect unusual activities[10][15].
Research Avenues
Several intriguing research avenues in Computer Vision include:
Object Detection and Tracking: The development of algorithms that identify and
track objects within images and videos is crucial for applications in surveillance and
automotive safety[11][16].
Image Captioning: This area bridges the gap between visual and textual comprehension
by generating descriptive captions for images, which is beneficial for visually
impaired individuals and enhances multimedia indexing[10][17].
Key Techniques
Among the various techniques employed in Computer Vision, the following are
particularly significant:
Convolutional Neural Networks (CNNs): These deep learning models are designed
to process and classify images effectively, mimicking the way the human brain
recognizes patterns[18][15].
Image Segmentation: This technique involves partitioning an image into multiple
segments to simplify its analysis[10][12].
Feature Extraction: This process identifies and extracts important features from
images to aid in classification and recognition tasks[18][15].
Challenges
Despite its advancements, Computer Vision faces several challenges, including:
Motion Planning: Ensuring accurate object tracking and recognition in dynamic
environments remains a complex problem[14][18].
Sensor Integration: Combining data from various sensors to enhance the reliability
of computer vision systems poses significant technical hurdles[11][13].
Human-Robot Interaction: Developing intuitive interfaces that facilitate interaction
between humans and computer vision systems is crucial for applications in robotics[
14][18].
Through ongoing research and technological advancements, Computer Vision continues
to evolve, presenting new opportunities and challenges in the realm of
Artificial Intelligence.
Robotics
Robotics is a significant branch of artificial intelligence (AI) focused on the design,
construction, operation, and utilization of robots. This field combines engineering
and computer science to develop machines that can perform tasks autonomously
or semi-autonomously.
Applications of Robotics
Robotics has a wide range of applications, which can be categorized as follows:
Manufacturing Automation
In manufacturing, robots are commonly used for tasks such as assembly, welding,
painting, and packaging. Their precision and ability to work tirelessly make them
essential for improving efficiency and reducing production costs[18][19].
Medical Robots
Robots play a crucial role in healthcare, assisting in surgeries, rehabilitation, and
patient care. Surgical robots allow for minimally invasive procedures, enhancing
precision and recovery times[20].
Exploration
Robots are also utilized for exploration in challenging environments, including space
and underwater. They can gather data, conduct experiments, and carry out missions
that would be too dangerous for human beings[21].
Service Robots
Service robots are increasingly being deployed in environments such as hotels,
restaurants, and homes, performing tasks such as cleaning, delivery, and customer
assistance. This application reflects the growing trend of automation in everyday
life[22].
Definition and Overview
Robotics involves creating machines that can carry out various tasks, often mimicking
human actions or performing operations in environments that may be hazardous or
difficult for humans. These robots can be programmed to perform specific functions,
making them invaluable in a variety of settings, including industrial, medical, and
service applications[5][23].
Key Technologies and Components
Robotics integrates various technologies and components, including:
Actuators
Actuators are the mechanisms that enable robots to move and manipulate objects.
They convert energy into motion and can be found in various forms such as electric
motors and hydraulic systems[24].
Sensors
Sensors allow robots to perceive their environment. They can detect obstacles,
measure distances, and recognize patterns, enabling robots to navigate and interact
effectively[25].
Control Systems
Control systems are essential for managing the behavior of robots. They include
algorithms that determine how robots respond to sensor inputs and execute tasks,
ensuring accurate and efficient operation[26].
AI Algorithms
AI algorithms are increasingly being integrated into robotic systems, enabling them to
learn from experiences, adapt to new information, and make decisions autonomously.
This development is driving advancements in robotics, enhancing their functionality
and versatility[17].
Challenges in Robotics
Despite its advancements, robotics faces several challenges:
Motion Planning
Determining the most efficient path for a robot to navigate its environment is complex,
especially in dynamic settings where obstacles may change[27].
Sensor Integration
Integrating data from various sensors to create a coherent understanding of the
environment remains a significant hurdle in robotics[28].
Human-Robot Interaction
Designing robots that can effectively communicate and collaborate with humans is
essential, particularly in applications where safety and teamwork are critical[29].
Neural Networks
Neural networks are computing systems inspired by the biological neural networks
found in animal brains. They are a fundamental component of artificial intelligence
(AI) and machine learning (ML), enabling machines to perform complex tasks by
learning from data. Deep learning, a subset of ML, utilizes multi-layered neural
networks to model intricate patterns in large datasets[5][14].
Key Concepts
Several key concepts underpin the operation of neural networks:
Backpropagation: This algorithm is used for training neural networks, allowing them
to adjust their weights based on the error of their predictions[5].
Convolutional Networks: These networks are particularly effective for processing
grid-like data, such as images. They apply filters to capture spatial hierarchies in
data[14].
Recurrent Networks: These networks are designed to recognize patterns in sequences,
making them suitable for tasks involving time series data or natural language
[14].
Technologies
Various technologies support the implementation of neural networks, including:
TensorFlow: An open-source library developed by Google, TensorFlow facilitates the
creation and training of neural networks for various applications[5].
PyTorch: Developed by Facebook, PyTorch provides a flexible platform for deep
learning and is particularly favored for research purposes[5][14].
Keras: This high-level neural networks API runs on top of TensorFlow and allows for
easy and fast prototyping of deep learning models[5][14].
Applications
Neural networks have a wide range of applications across various domains:
Image Recognition: They are used extensively in facial recognition and object detection
technologies[14][30].
Speech Recognition: Neural networks play a crucial role in converting spoken language
into text and understanding human speech patterns[14][30].
Natural Language Processing: These networks help in understanding and generating
human language, facilitating tasks such as translation and sentiment analysis[5][30].
Game Playing: Neural networks have been successfully applied in developing AI that
can play complex games, demonstrating advanced strategic thinking[5][30].
Advantages
The advantages of neural networks include:
Consistency: Once trained, neural networks can consistently produce results, making
them reliable for various tasks[14].
Availability: They can operate continuously without fatigue, allowing for 24/7 application
in critical areas such as healthcare and finance[14][30].
Expertise Replication: Neural networks can replicate the decision-making abilities of
human experts, making them valuable in fields requiring specialized knowledge, such
as medical diagnosis and financial forecasting[5][30].
Deep Learning
Deep learning is a subset of machine learning that employs neural networks with
multiple layers to model complex patterns in large datasets. It is particularly effective
in handling unstructured data such as images, text, and audio, thereby enabling significant
advancements in fields like computer vision and natural language processing
(NLP) [4][2].
Applications of Deep Learning
Deep learning techniques have found widespread application across various industries.
In computer vision, for instance, deep learning is utilized for tasks such
as image recognition, object detection, and image segmentation. These techniques
enhance capabilities in sectors including healthcare, where they assist in diagnosing
diseases from medical images, and autonomous vehicles, which rely on accurate
visual understanding to navigate [31][8].
In the realm of NLP, deep learning facilitates the development of sophisticated models
for tasks like sentiment analysis, machine translation, and question-answering
systems. Such models are designed to grasp nuances in human language, thereby
improving user interactions in applications ranging from chatbots to automated
customer support [8][32].
Techniques in Deep Learning
Deep learning leverages various architectures, with the most notable being convolutional
neural networks (CNNs) and recurrent neural networks (RNNs). CNNs
excel in processing grid-like data, making them ideal for image analysis, while RNNs
are suited for sequential data, such as time series or text. Additionally, generative
adversarial networks (GANs) are employed for generating new data samples that
resemble existing data, showcasing deep learning's versatility [4][33].
Future Trends
As the field of deep learning continues to evolve, research is increasingly focusing
on improving the interpretability and efficiency of deep learning models. Efforts are
underway to develop algorithms that require less labeled data for training, as well as
those that can operate in real-time on devices with limited computational resources.
This shift is driven by the demand for AI solutions that are not only powerful but also
practical and accessible in everyday applications [31][34].
Deep learning remains a critical area of study within artificial intelligence, representing
both current capabilities and future potential in developing intelligent systems
that enhance various aspects of human life.
AI Ethics
AI ethics encompasses the moral principles and frameworks guiding the development
and application of artificial intelligence technologies. As AI systems become
increasingly integrated into various sectors, ethical decision-making becomes critical
to align these technologies with societal values and expectations[9][17].
Ethical Decision-Making Frameworks
Developing robust ethical decision-making frameworks is essential to navigate the
complexities of AI applications. These frameworks aim to imbue AI with capabilities
that reflect ethical considerations, thereby ensuring responsible innovation and use-
[9][35]. The establishment of such frameworks is not only crucial for safeguarding
individual rights but also for enhancing public trust in AI technologies[36][20].
Key Challenges in AI Ethics
Artificial General Intelligence (AGI)
The potential emergence of Artificial General Intelligence (AGI) raises profound
ethical questions. Discussions surrounding AGI focus on its capability to emulate
human-like intelligence and the associated implications and challenges[35][26]. Ensuring
that AGI systems operate within ethical boundaries is paramount to prevent
adverse societal impacts.
AI and Creativity
The intersection of AI and creative domains, such as art and music, highlights ethical
considerations regarding authorship, originality, and the role of human creativity in
collaboration with machines[35][17]. As AI systems contribute to creative processes,
understanding the ethical dimensions of these interactions becomes increasingly
important.
Regulatory Frameworks
Researching the ethical dilemmas that arise in the context of AI's evolution is crucial
for establishing effective regulatory frameworks. These frameworks are designed to
address concerns such as biased data, transparency, and the explainability of AI
systems, ultimately aiming to protect users and foster trust in AI technologies[17][36].
Best Practices for Ethical AI
To promote ethical AI, organizations are encouraged to adopt best practices, such
as appointing dedicated ethics leaders and maintaining a holistic perspective that
encompasses both legal and moral dimensions[20][5]. These practices help ensure
that AI technologies not only comply with legal standards but also adhere to ethical
principles that benefit society as a whole.
Future Considerations
As AI continues to advance, ongoing research and dialogue surrounding ethical
issues will remain vital. Enterprises must integrate ethical considerations into their
strategic frameworks, viewing them as opportunities for building trust with customers
and regulators rather than merely as compliance requirements[5]. The future of AI
ethics will depend on collaborative efforts among researchers, business leaders, and
regulators to create responsible and impactful AI solutions.
Autonomous Systems
Definition and Functionality
An autonomous system is a technology capable of sensing its environment and
making decisions with minimal or no human intervention. One prominent example
of an autonomous system is the autonomous car, which uses a vast array of sensors
to continuously gather data about its surroundings. This data is processed by the
vehicle's autonomous driving computer system, enabling it to navigate safely through
various traffic situations[37][16][8]. To operate effectively, these systems undergo
extensive training to interpret the collected data and make appropriate decisions in
a wide range of scenarios[37][25].
Ethical Considerations
As autonomous systems, particularly autonomous vehicles, become more prevalent,
ethical dilemmas surrounding their decision-making capabilities arise. For instance,
when faced with an emergency, an autonomous vehicle may need to make a moral
decision, such as choosing whether to brake suddenly to avoid hitting a jaywalker,
potentially putting the occupants of the vehicle at risk[16][15]. This shift in risk
assessment reflects the complex moral landscape that developers and policymakers
must navigate as they design and implement autonomous technologies.
Multimodal Capabilities
Modern autonomous systems increasingly leverage multimodal AI, which integrates
multiple forms of data (textual, visual, etc.) to enhance decision-making and functionality.
For instance, in the field of medicine, AI is now capable of merging various
data types to provide comprehensive insights into patient health, demonstrating
the potential of multimodal approaches within autonomous systems[21][38]. Such
capabilities not only improve the effectiveness of autonomous systems but also
expand their application across diverse sectors.
Future Developments
The landscape of autonomous systems continues to evolve, with growing interest in
agentic AI models that can operate independently within defined parameters. These
models, such as Salesforce's Agentforce, illustrate the trend toward systems that
can manage workflows and perform tasks autonomously while adapting to real-time
changes in their environment[18]. As the technology matures, the focus will likely
shift toward ensuring human oversight and addressing the ethical implications of
autonomous decision-making in critical areas.
AI in Healthcare
Artificial Intelligence (AI) is rapidly transforming the healthcare industry by enhancing
diagnostic accuracy, optimizing treatment selection, and streamlining operational
processes. The integration of AI into healthcare systems is proving to be a valuable
asset in improving patient outcomes and reducing costs.
Benefits of AI in Healthcare
AI technologies contribute significantly to cost reduction in healthcare by optimizing
resource allocation and detecting fraudulent claims, which results in substantial
financial savings across the industry[10][39]. Specifically, AI can assist in revenue
cycle management and improve health outcomes through insights derived from
electronic health records (EHR) and patient data[40].
Improved Diagnostics and Decision-Making
AI enhances medical diagnosis and clinical decision-making by improving data
accessibility across healthcare systems. By analyzing large datasets, AI tools can
identify patterns that might elude human practitioners, thereby accelerating diagnoses
and fostering collaborative healthcare delivery[31][41]. This capability not only
leads to time savings but also minimizes human errors, making the healthcare system
more efficient[42].
Support for Healthcare Research
In addition to its role in clinical settings, AI plays a pivotal role in healthcare research.
It supports innovations such as precision medicine, drug discovery, and clinical trials
by identifying eligible patients, predicting dropout rates, and optimizing trial designs.
Notable companies in this space include Deep Genomics and BenevolentAI, which
focus on AI-driven drug discovery, as well as Antidote and TrialSpark, which develop
AI-powered clinical trial matching platforms[43][29].
Future Directions
The potential of AI to revolutionize healthcare is immense. Its applications range
from personalized medicine and optimized medication dosages to enhancing population
health management and providing virtual health assistants[44][45]. As AI
technologies continue to evolve, they are expected to play an increasingly integral
role in shaping patient care, enhancing medical training, and advancing healthcare
research.
Challenges and Considerations
Despite its potential, the integration of AI in healthcare also raises legal, ethical,
and risk-related concerns that must be addressed to ensure responsible implementation[
46][47]. These challenges will require ongoing dialogue among stakeholders,
including healthcare providers, policymakers, and technology developers, to navigate
the complexities of AI in healthcare effectively.
AI in Finance
Artificial intelligence (AI) is increasingly transforming the finance industry by enhancing
data analytics, performance measurement, forecasting, and customer service
capabilities. The integration of AI technologies, particularly generative AI, has led to
significant improvements in various aspects of financial operations.
Benefits of Generative AI in Finance
Generative AI has been identified as a critical tool in improving financial conditions
across several dimensions:
Improved Financial Metrics: The implementation of generative AI solutions has resulted
in a 33% faster budget cycle time, a 43% decrease in uncollectible balances, and
a 25% reduction in expenses for every invoice processed[48][49][50].
Enhanced Research Efficiency: According to EY, investment banking benefits from
more efficient research and financial modeling, while corporate and small to medium-
sized businesses (SMBs) see improvements in risk management and business
lending. Consumer banking experiences enhanced service delivery and customer
interactions due to AI advancements[51][49].
Business Partnering: Generative AI facilitates more accurate insights and forecasts
by swiftly analyzing extensive financial datasets. This capability fosters collaboration
between finance teams and other business units, generating detailed reports and
responses that support better decision-making processes[48][49].
Risk Management Applications
Generative AI also plays a crucial role in risk management:
Stress Testing: By simulating extreme market conditions that have not been observed
in historical data, generative AI enables financial institutions to prepare for rare but
potentially impactful events[48][50].
Credit Risk Modeling: AI can create synthetic borrower profiles to test and enhance
the robustness of credit risk models, thereby improving the accuracy of credit scoring
and default predictions[49][50].
Anomaly Detection: Generative AI enhances fraud detection capabilities by analyzing
patterns and identifying suspicious activities with greater accuracy. For instance,
Mastercard utilized generative AI to analyze transaction data, which resulted in a
doubled detection rate of compromised cards and significantly reduced false positives
in fraud detection[52].
Sources:
[31]: AI Research Trends
ComentĂĄrios